Large datasets of entire genomes will help identify disease subtypes and optimize treatments
PDF Download
- Medical big data analysis
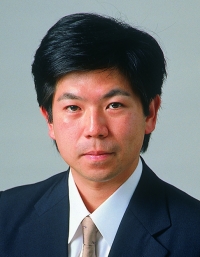
Tatsuhiko Tsunoda
Professor of Medical Science Mathematics at TMDU
Profile
Dr. Tsunoda graduated from the University of Tokyo, where he studied elementary particle physics and computer science. He obtained two PhDs -- in 1995 in Engineering, and in 2007 in Medicine. He started his career as Assistant Professor at Kyoto University in 1995 and then became Research Associate and Assistant Professor at the University of Tokyo. In 2000, he moved to RIKEN, where he continues to lead the Research Group for Medical Science Mathematics, RIKEN Center for Integrative Medical Sciences. He became Professor of Medical Science Mathematics at TMDU in 2015.
A: Ever since scientists started to unravel the secrets of the human genome, a time was predicted when differences in individual genes, lifestyles, and environments could be taken into account when selecting treatment strategies. Precision medicine aims to achieve just that, by optimizing prevention or therapeutic interventions to suit the needs of groups of similar individuals rather than taking a “one size fits all” approach. This is particularly important for cancer therapy, which has traditionally relied on treating the type of cancer based on the location, e.g. tissue, of the tumor in the body. However, tumors within the same region may derive from different mutations and mechanisms. This causes the tumors to respond differently to treatment. “Omicsprofiling” teases these differences apart, and builds up an outline of patients with respect to differences in their genetics (somatic and germline variations, including copy number and structural variations), gene expression regulations, protein expression changes and modifications, and the production of small molecules and autoantibodies. These outlines not only help us understand disease development and categorize subtypes, but also identify disease “biomarkers” that can be used to predict patient outcomes. Worldwide collaborative efforts between clinicians and scientists have enabled the coordination of such information for a huge number of patients, but this big data requires careful mathematical analysis. To this end, I have proposed a novel bottom-up statistical method that considers the many differences among samples, and can be combined with a top-down analysis for biomarker exploration.
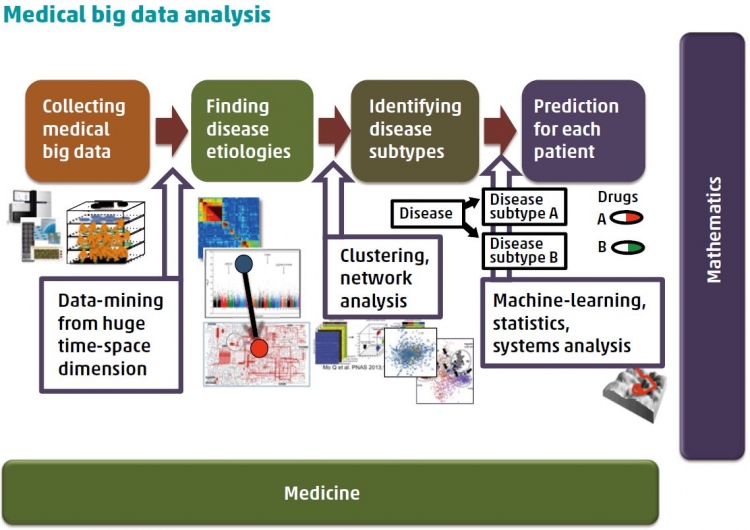
focus on this disease and what did you discover?
A:As the fifth most common cancer worldwide, liver cancer places a huge burden on public health. It is mainly caused by infections of hepatitis B and C viruses, but its numerous other causes include alcohol intake and exposure to liver carcinogens. Several genes have been identified that are mutated in 30%–60% of liver cancer cases, as well as many that are mutated in a tiny number of cases. However, as with all genetic analysis, the greater the sample size the more statistical punch it can pack. We therefore looked at the entire genomes of 300 Japanese patients with liver cancer, mostly hepatocellular carcinomas.
Each mutation that occurs during cancer development leaves its mark on the genome, and these “mutational signatures” have previously been identified in liver cancer patients of other ethnicities. The signatures we detected that associate with hepatocellular carcinoma in Japanese patients did not match those from French or Chinese studies, suggesting that different populations undergo a diff erent set of mutations in their development of cancer. More mutations were found in older patients, and those who smoked or had larger tumors. They were also more common in parts of the genome that replicated themselves late in the cell cycle, possibly because these are less accessible to DNA repair mechanisms. Driver mutations confer a growth advantage on cancer cells, and we identified several new driver genes associated with liver cancer, including those involved in liver metabolism, DNA repair,and DNA chromatin remodeling. We showed that DNA rearrangements in the form of deletions and duplications aff ected the expression of cancer-related genes, while sections of DNA that do not code for proteins (such as promoters or other regulatory regions) were also repeatedly mutated.
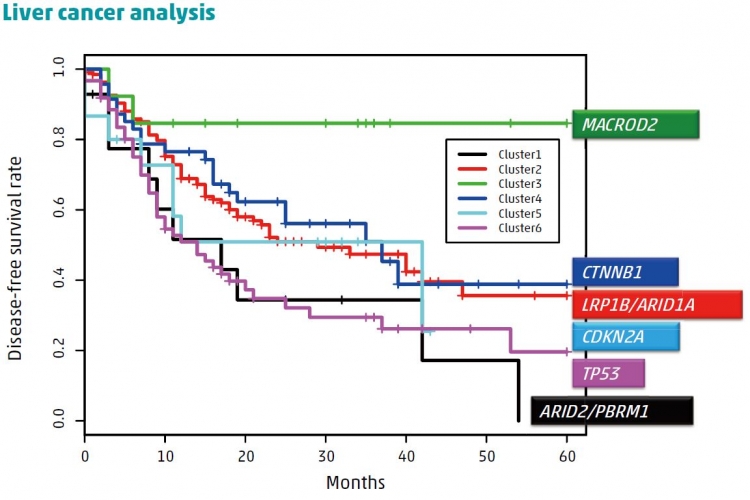
A: Because our work looked at the entire genomes of patients, it was common for their mutation distributions to be sparse. To overcome this problem, we used a network-based stratification approach that relied on the fact that mutations causing the same disease often occur in genes that are in close proximity and within a network of interactions between proteins.
A statistical technique based on the bottom-up approach was used to divide the total population into clusters of patients with mutations in similar network regions. We then used further statistical analyses to compare the length of time that patients within clusters survived without disease recurrence to help understand the biological importance of different mutations and their effects on patient survival.
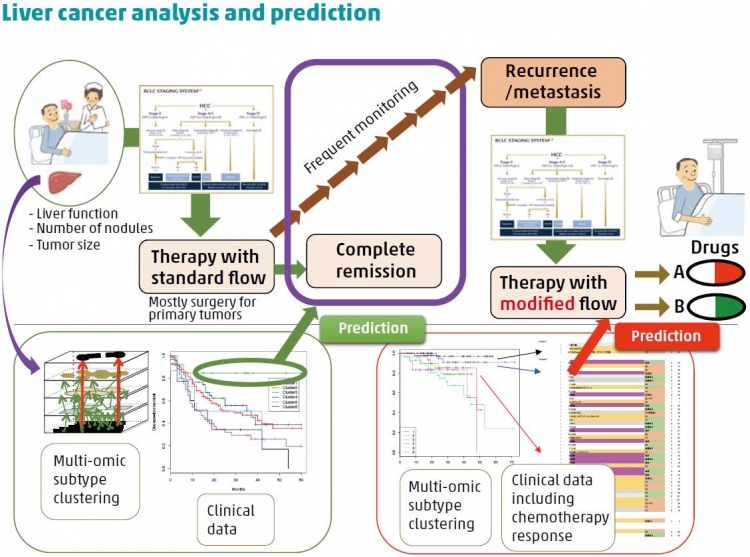
A: Some of the Japanese mutation signatures that we identified, as well as some individual mutations, were more likely to occur if the patients smoked, drank alcohol, or were infected with hepatitis B or C. This strongly shows the importance of gene–environment interactions on the development of disease. Using the statistical technique mentioned above, we identified six clusters of patients, with each cluster sharing similarities such as mutations in particular driver genes. When taking into account
other factors like age, sex, and whether the patients had undergone surgery, we found that patients in two clusters had a significantly lower chance of survival while those in another cluster had a significantly better chance of recurrence-free-survival than individuals in other clusters. These findings can be used to target patients who will best respond to particular therapies or interventions.