Big Data and Statistical Genetics
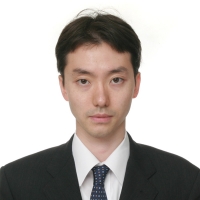
Yukinori Okada,
Junior Associate Professor of Human Genetics and Disease Diversity
A major challenge in human genetics is to devise a systematic strategy to integrate disease genome Big Data with diverse datasets to provide insight into disease pathogenesis and to guide drug discovery. After two years of experience as a post-doc researcher at Harvard Medical School, I started my career as a tenure-track faculty member at TMDU. The research theme of our group is to empirically prove that in silico approaches based on Statistical Genetics can contribute to this challenge. We constructed an in silico bioinformatics pipeline to systematically integrate the identified rheumatoid arthritis (RA) risk genetic loci with a variety of biological, medical, and epidemiological databases. We demonstrated that RA-risk genetic loci are significantly enriched with genes that are the target of therapies currently approved for RA treatment. Our analysis further suggested that drugs approved for other disease indications may be repurposed for the treatment of RA (e.g., CDK4/CDK6 inhibitors currently used for treating cancer).
A visionary project applying a cognitive computing system to disease genome BIG DATA has also been launched to develop a path to drug discovery. We have recently developed a novel genetic analytical framework named “HLA imputation method”, which can computationally estimate high-resolution HLA gene polymorphisms. Comprehensive HLA gene analysis by the HLA imputation method successfully elucidated risk biomarkers that contribute to both the onset and development of autoimmune diseases, such as Graves’ disease. Together, our studies provide empirical evidence that Statistical Genetics can provide important information for human diseases, including novel therapeutic targets and drug discovery, in the era of Big Data. (doi:10.1038/nature12873).
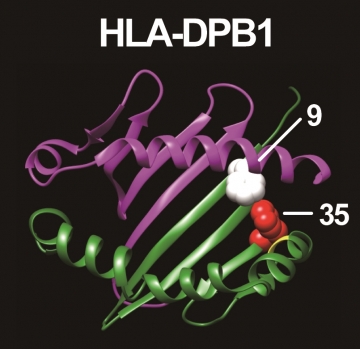
HLA-DPB1 amino acid positions with Graves’ disease risk