Research
Advanced neuroimaging and development of multimodal learning
Neuroimaging studies to visualize pathogenesis, neural networks and therapeutic effects
We are using advanced neuroimaging studies to visualize disease processes in vivo which are actually occurring in a patient’s brain. We perform non-invasive multimodal neuroimaging studies with different MRI sequences to evaluate multifaceted structures and functions of the brain.
The following methods were implemented to visualize and quantify brain structure and activity as well as the neural network. (1) Functional connectivity is used to specify anatomical areas where neural activities are chronologically synchronized during the resting state with functional MRI. These areas are supposed to be functionally connected and work as a network (Figure A); (2) Structural connectivity is used to identify anatomical connections between grey matter by using diffusion tensor imaging (Figure B); and (3) Regional blood perfusion is used to evaluate local synaptic activity by using arterial spin labelling (Figure C). We also use cutting-edge neuroimaging methods to detect bleeding and grey matter damage.
To date, we have reported the following : (a) alteration of the functional network for motor function (OHBM, 2015); (b) brain activation patterns using a working memory task (OHBM, 2018, Figure D) as well as grey and white matter and perfusion changes during the progression of cognitive impairment in Parkinson disease (Hum Brain Mapp, 2012); (c) microstructural alteration in multiple system atrophy (ISMRM, 2013) and in idiopathic normal pressure hydrocephalus (AJNR, 2011, 2012, Figure E); and (d) structural connectivity in the spatial attention network underlying left hemispatial neglect (Brain Imaging and Behavior, 2018, Figure F).
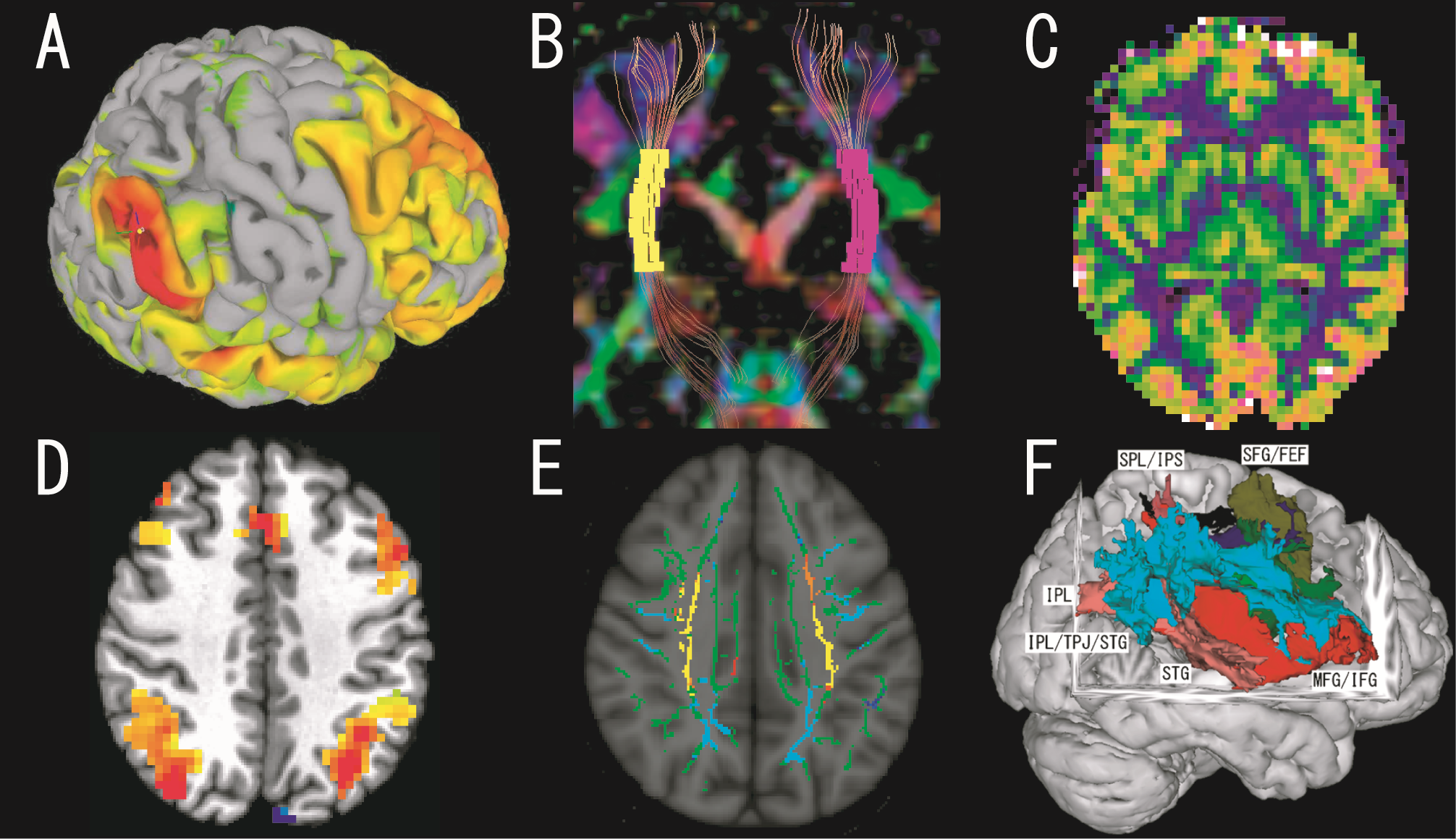
Currently, we are performing neuroimaging studies for Parkinson's disease (PD), atypical parkinsonian syndromes, amyotrophic lateral sclerosis (ALS), idiopathic normal pressure hydrocephalus (iNPH), multiple sclerosis (MS) and neuromyelitis optica (NMO). Furthermore, we are conducting gait analysis and comprehensive cognitive assessment for same patients to better interpret neuroimaging results.
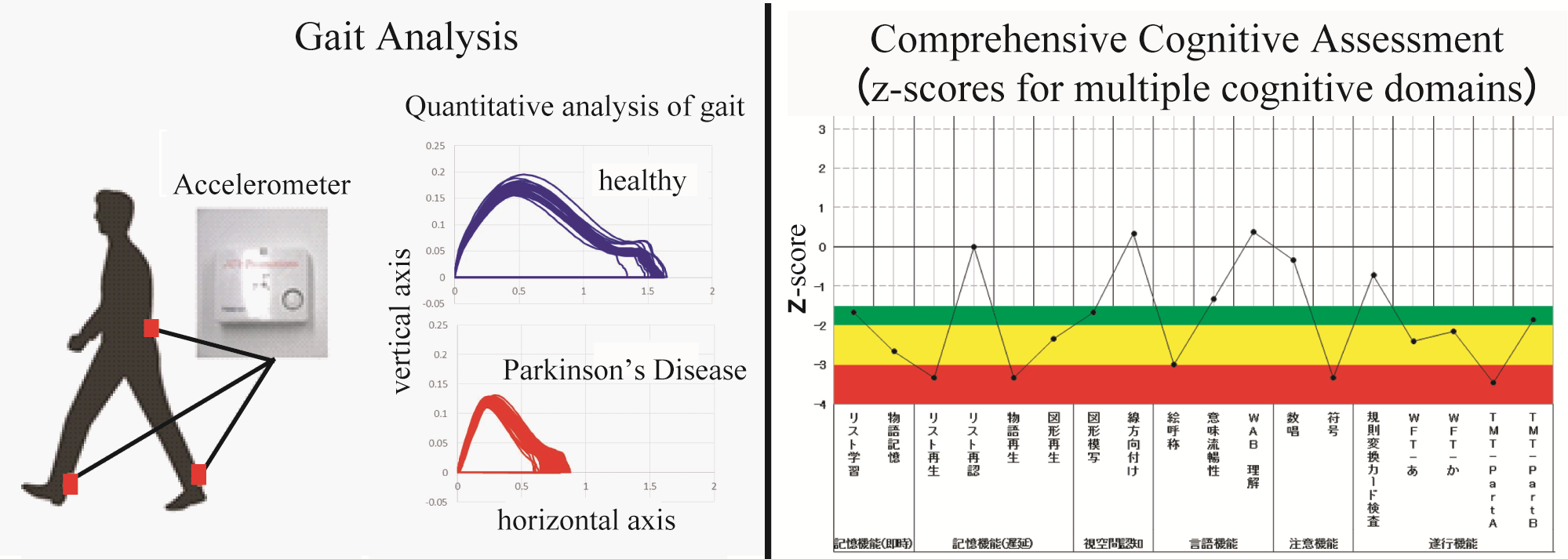
Parkinson's Disease and Atypical Parkinsonian Syndrome
Patients with Parkinson's disease (PD) and atypical parkinsonian syndromes develop motor symptoms such as bradykinesia, gait disturbance, rigidity, resting tremor and postural instability due to dopaminergic denervation. Motor symptoms are often responsive to dopaminergic medications, especially in PD. Despite extensive research, the neural correlates of motor symptoms and the exact mechanism how dopaminergic medications work remain unknown. Here, we aim to specify the neural underpinnings of motor symptoms and how dopaminergic medications modulate neural networks by using multimodal neuroimaging and gait analysis. In addition, we are also trying to establish novel rehabilitation methods to induce neural plasticity improving gait disturbance by applying multi-modal sensory inputs while walking.
This research will help us to better understand the pathogenesis of parkinsonism and to establish reliable biomarkers which are useful for making accurate diagnoses and evaluating therapeutic effects.
Amyotrophic Lateral Sclerosis
Amyotrophic lateral sclerosis (ALS) has been known as a motor neuron disease which selectively damages motor systems, in particular the primary motor cortex and spinal anterior horns. However, recent studies reveal that ALS affects not only the motor cortex but also others brain areas, such as the basal ganglia and the frontal lobes. In contrast, conventional MRI has limited sensitivity to detect damage in the brain, and clinicians often find it difficult to make a diagnosis of ALS. Here, we aim to visualize structural and functional alterations in the brains of ALS patients by using multi-modality neuroimaging for motor and cognitive neural networks, cortical thickness and microstructure in white matter as well as electrophysiological studies and gait analysis.
This study will enable us to have objective methodology to evaluate brain damage in ALS patients, leading to accurate diagnosis and correct estimation of disease stage, progression, and therapeutic effect.
Idiopathic Normal Pressure Hydrocephalus and its Related Disorders
Idiopathic normal pressure hydrocephalus (iNPH) is a clinical entity characterized by the clinical triad of gait disturbance, urinary incontinence, and dementia with enlarged lateral ventricles. Although iNPH has been emphasized as a potentially reversible cause of gait disturbance and dementia, the neural correlates of their symptoms remain unclear. We aim to specify the neural underpinning of iNPH symptoms by comparing neuroimaging and gait analysis before and after cerebrospinal fluid (CSF) removal test.
There are no established pathological hallmarks of iNPH, and the symptoms of iNPH patients are often worsened even after shunt operation. Several case studies reported that patients with ante-mortem diagnosis of definite iNPH had different post-mortem diagnosis such as progressive supranuclear palsy. According to these studies, we hypothesize that there is an iNPH like tap-responsive state secondary to neurodegenerative diseases. Currently, we are conducting the prospective studies in which we perform CSF removal test for patients with neurodegenerative disorder to determine whether they respond or not by evaluating gait, cognitive functions, and advanced neuroimaging before and after CSF removal test.
This study will help us to better understand the true epidemiology and neural correlates of iNPH and CSF removal test-responsive neurodegenerative disorders, leading to accurate diagnosis and proper treatments.
Multiple Sclerosis (MS) and Neuromyelitis Optica (NMO)
Multiple sclerosis (MS) is chronic inflammatory disease in the central nervous system (CNS), often affecting young adults. MS causes a variety of CNS symptoms, and CNS lesions are accumulated together with clinical and subclinical relapses. Although fundamental treatments have not been established yet, an increasing number of disease-modifying treatments (DMT) is available to control the disease activity of MS. However, the responses of MS patients for each DMT are highly variable depending on each subject, and it is difficult to accurately assess individual response in a short period of time.
Neuromyelitis optica (NMO) is similar to MS, but an independent chronic inflammatory CNS disease. NMO affects not only the optic nerves and spinal cord, but also the brain. CNS lesions are accumulated by symptomatic and subsymptomatic relapses, impairing the daily life of patients. Although immunosuppressive therapy is often used to control disease activity of NMO, it is difficult to properly evaluate NMO's disease activity and responsiveness to immunosuppressive therapy in a short period of time.
Currently, we are trying to establish reliable surrogate markers of disease activity of MS or NMO, which should be simple and available in clinical practice. For this objective, we are building a database by performing detailed clinical and radiological assessments for patients with MS or NMO with multi-modal advanced neuroimaging, gait analysis, and cognitive assessment.
This study will enable us to accurately assess the disease activity of MS or NMO as well as individual response to therapies, leading to less relapses and preventing progression of symptoms.
Network analysis as a brain system: Graph theory
We analyze the entire brain as a network. Therefore, we evaluate structural and functional connectivity among regions of the brain using three-dimensional T1(3DT1)-weighted images, diffusion tensor images, and resting-state functional MRI images.
We employ graph theory to analyze brain network. Graph theory consists of a mathematical analysis of geometric figures, (graph), which is created by dividing the brain into hundreds of regions (nodes) and connecting the regions with lines (edges). The graph theory enables us to quantitatively evaluate brain networks in terms of functional separation, functional integration, or network clustering, etc.
In neurodegenerative diseases, pathogenic proteins aggregate in specific brain regions, spreading to certain areas and generating specified propagation patterns. Therefore, it is speculated that the structural pattern of the whole brain reflects spreading and aggregation of certain pathogenic proteins. Specifically, we analyze the structural network of the brain using 3DT1-weighted images of patients with Alzheimer's or Parkinson's diseases using the graph theory to identify degenerated structural networks. We believe that the aberrant network pattern reflects propagation and aggregation of disease-specific proteins such as amyloid, tau, and α-synuclein; additionally, we try to verify it.
We apply network analysis for multimodal neuroimaging data to better understand pathophysiology and alteration of brain networks before/after treatments, and to integrate neuroimaging data to multimodal machine learning, as described below.
Development of multimodal machine learning technology
We are developing multimodal machine learning technology to integrate advanced neuroimaging data (neural networks, structural information of white and gray matter), gait analysis, motion video analysis and comprehensive cognitive examinations, which enables us to discover the novel scientific findings, pathogenesis of the disorder, and potential therapeutic effects of drugs.
Understanding pathogenesis by deep learning for advanced neuroimaging
Advanced neuroimaging contains a huge amount of information such as the extent of brain damage which precedes the pathological state prior to(a) the onset of symptoms, and (b) possible therapeutic effects. Recently, artificial intelligence (AI) has developed rapidly; in particular, the accuracy of image classification using deep learning has improved dramatically. We plan to develop a novel deep learning system that enables us to understand the pathological states of patients from his/her advanced neuroimaging. While several problems need to be solved to establish the deep learning system, we are trying to tackle them by the following strategies.
1) A limited number of data is available: Transfer learning
Deep learning handles complex imaging and enables us to accurately interpret subsequent images. In general, training deep learning requires a large amount of data to achieve high performance. However, it is not easy to collect such a sizeable amount of data in one medical institute. To solve this problem, we use a technique called “transfer learning”, which refers to applying a pre-trained model for learning our neuroimaging data together with learning other independent data sets.
2) Visualization of deep learning: Grad-CAM
While deep learning using convolutional neural networks successfully recognizes images with high performance, the judgment process of deep learning is unclear. Here, we use the Gradient-weighted Class Activation Mapping (Grad-CAM) technology to visualize the anatomic areas which deep learning has focused on to make the decision. Then, we can determine the specific characteristic finding of advanced neuroimaging which may help classify the disease.
Prediction of diagnosis by machine learning of gait analysis
To date, previous studies for gait analysis mainly evaluated typical parameters of gait such as step length and walking speed. On the other hand, our gait measurement system collects more multifaceted data regarding gait; we believe that the comprehensive data reflect pathological conditions. Our gait analysis generates more than 50 parameters of gait. On the other hand, the relationship between the parameters is complicated and statistical analysis is not easily applicable. Thus, we need to apply the machine learning algorithm to analyze gait parameters to derive characteristic features of gait reflecting each disease.
Gait parameters can be classified into three categories: mean, variabilities, and left-right asymmetries of each parameter. The optimal learning model (support vector machine, random forest, etc.) may differ depending on each parameter. Thus, the best learning model is used for each data, and the conclusion can be made by majority voting.
Multimodal machine learning integrating advanced neuroimaging, gait analysis, motion video analysis and comprehensive cognitive examinations
We are aiming to develop multimodal machine learning that can discover novel findings by integrating advanced neuroimaging, gait analysis, motion video analysis, and comprehensive cognitive examinations. We plan to predict motor and cognitive functions from advanced neuroimaging by performing “supervised learning” in which gait analysis and cognitive function are handled as labeled data. We wish to extract clinically important information such as degenerated areas of the brain, pathogenesis, and the therapeutic effects from advanced neuroimaging.
Furthermore, we intend to predict damaged brain systems from comprehensive cognitive examinations, or to predict impaired brain systems and cognitive functions from gait analysis or motion video analysis alone. We believe that developed multimodal learning will contribute to making more accurate diagnosis, evaluating treatment effects, discovering novel scientific findings, and predicting other modality data from one other modality data.
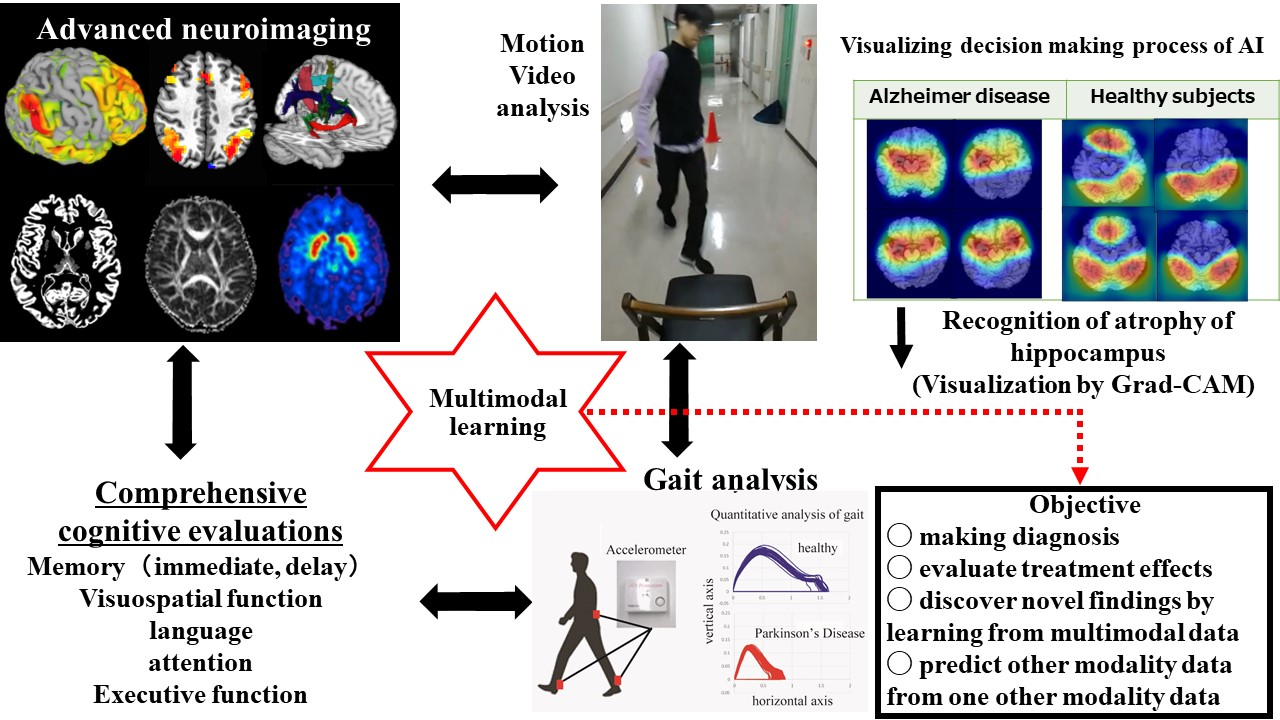